Data Analyst Syllabus
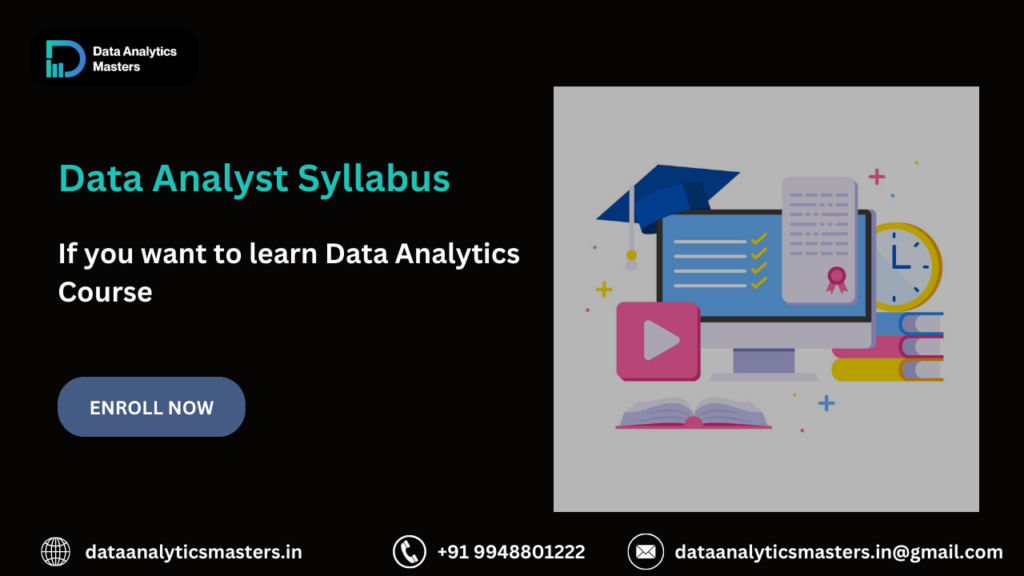
An overview of Data Analyst Syllabus
In this blog, I’ll guide you about the key topics covered in a typical Data Analyst Syllabus.
- This syllabus is designed to equip students and professionals with the essential skills needed for effective data analysis.
- Whether you’re a beginner or looking to level up your skills, the syllabus includes everything you need to know to become proficient in data analysis, including data cleaning, statistical techniques, data visualization, and much more.
- I’ll also cover the tools you’ll be learning to use, like Excel, SQL, Python, and Tableau, which are critical for the job.
The syllabus is thoughtfully structured to facilitate a smooth learning curve, especially for beginners:
Introduction to Data Analysis: This section explains what data is, how to gather it, and how to understand and organize it. You’ll start with foundational concepts that set the stage for more complex topics.
Data Cleaning and Preparation: Techniques for handling missing data, removing outliers, and transforming data for analysis are covered. Starting with basic statistics and Excel ensures you grasp the essential skills before moving to more advanced tools.
Statistical Analysis: Understanding concepts like mean, median, variance, and probability for data interpretation. These basics are crucial as you progress to more complex analyses.
Data Visualization: Tools and techniques for presenting data in charts, graphs, and dashboards. Data visualization tools, such as Tableau, help you present your findings clearly and effectively.
Data Analytics Tools: Hands-on experience with tools like Excel, SQL, Python, R, and data visualization tools. As you advance, you’ll work with SQL and Python, building on the foundational skills from Excel.
Advanced Analytics: Learning machine learning concepts, predictive modeling, and AI in data analysis. This section builds upon the skills acquired in earlier modules, offering a deeper dive into advanced analytics.
Real-World Applications: Understanding business problems and applying analytics to solve them. The syllabus ensures that theoretical knowledge is reinforced with practical, hands-on exercises, preparing you to tackle real-world challenges confidently.
This structured approach ensures that you build a solid foundation before progressing to more complex topics, providing a comprehensive learning experience that is both theoretical and practical.
1. Introduction to Data Analytics
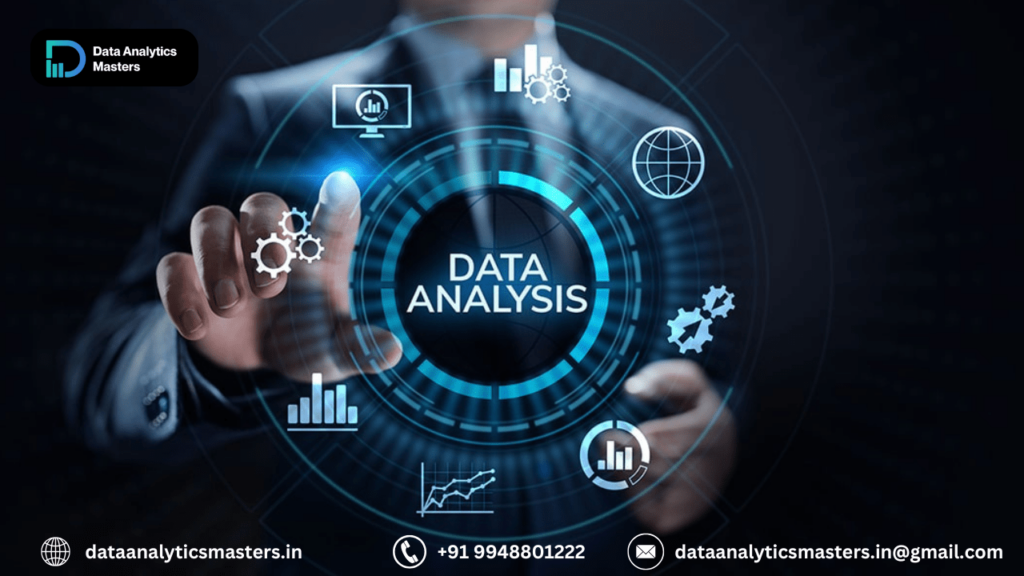
What is Data Analytics?
- Data analytics is the process of examining raw data to find patterns, trends, and insights that help in decision-making. It involves collecting, organizing, analyzing, and visualizing data to drive better business outcomes.
Importance of Data in Decision-Making
-
Data plays a crucial role in making informed decisions. Businesses use data to understand customer behavior, market trends, and operational efficiency. With accurate data analysis, companies can reduce risks, improve performance, and gain a competitive edge.
Types of Data Analytics
- Descriptive Analytics – Summarizes past data to understand what happened (e.g., sales reports, website traffic analysis).
- Diagnostic Analytics – Identifies reasons behind past trends (e.g., why sales dropped last month).
- Predictive Analytics – Uses data and AI to forecast future outcomes (e.g., predicting customer demand).
- Prescriptive Analytics – Recommends the best actions based on data insights (e.g., personalized marketing strategies).
Data Analytics vs Data Science vs Business Analytics
- Data Analytics is all about looking at data to find patterns and useful information that help make decisions.
- Data Science involves advanced techniques, including AI and machine learning, to build predictive models.
- Business Analytics applies data analytics specifically to business problems, helping organizations optimize operations and strategies.
2. Basics of Statistics and Probability
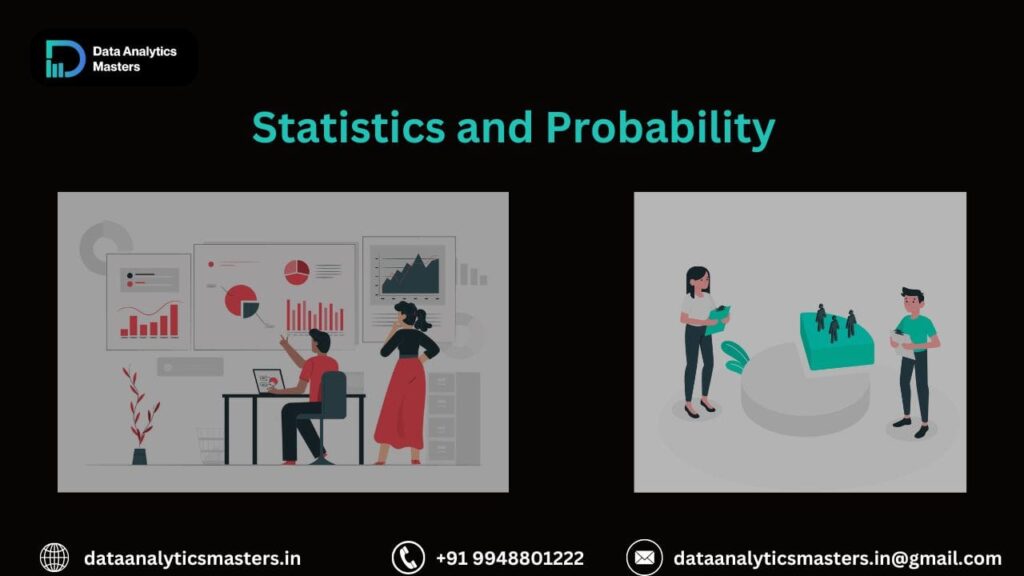
Measures of Central Tendency (Mean, Median, Mode)
These are important statistics that help summarize data:
- Mean: The average of all the numbers.
- Median: The middle number when the data is sorted.
- Mode: The number that appears most often.
They help in understanding the overall trend of data and are widely used in AI models for data preprocessing.
Measures of Dispersion (Variance, Standard Deviation)
These measures show how spread out the data is:
- Variance: The average difference from the mean, squared. Higher variance means the data is more spread out.
- Standard Deviation: The square root of variance, showing how much the data differs from the mean.
AI and machine learning models rely on these metrics to detect anomalies and assess data variability.
Probability Theory & Distributions
Probability helps us figure out how likely something is to happen. Common probability distributions include:
- Normal Distribution – Bell-shaped curve used in AI models for predictions.
- Binomial Distribution – Used when there are two possible outcomes (e.g., pass/fail, yes/no).
- Poisson Distribution – Models rare events (e.g., website crashes per day).
These distributions help in predicting future events and making decisions using AI.
Hypothesis Testing and Confidence Intervals
- Hypothesis Testing – A method to check if a claim about data is true (e.g., “Does a new ad increase sales?”).
- Confidence Intervals – A range of values within which a population parameter is likely to fall.
3. Excel for Data Analysis
Data Cleaning and Formatting
- Organizing raw data by removing duplicates, handling missing values, and standardizing formats.
- Ensures accuracy and prepares data for analysis in AI and machine learning models.
Formulas & Functions for Data Analysis
- Essential functions like SUM(), AVERAGE(), IF(), VLOOKUP(), and INDEX-MATCH for quick calculations.
- Used to automate repetitive tasks and analyze large datasets efficiently.
Pivot Tables & Pivot Charts
- Pivot Tables summarize large datasets by grouping, sorting, and filtering data dynamically.
- Pivot Charts provide interactive visualizations for better insights and trend analysis.
Data Visualization in Excel
- Creating charts (bar, line, pie, scatter) to present data in an easy-to-understand format.
- It helps AI make decisions by showing patterns and trends in a visual way.
Advanced Analytical Techniques
Excel extends beyond basic functionalities to solve complex analytical problems:
- Scenario Manager, Solver, and Goal Seek: These tools allow analysts to address both stochastic and deterministic problems, enhancing predictive capabilities.
- Implementing statistical methods like hypothesis testing and regression analysis to deepen insights and validate assumptions.
Excel Macros & Automation
- Using VBA (Visual Basic for Applications) to automate tasks that are done over and over again.
- Enhances efficiency in data processing, reducing manual effort and errors.
Leveraging Advanced Tools
For those looking to push the boundaries of business analytics, integrating Excel with advanced tools can amplify analytical power:
- Introduction to Power BI: This advanced tool provides dynamic reporting and data visualization capabilities, offering an expansive view of analytical insights.
4. SQL for Data Extraction and Management
Basics of Databases & SQL
- SQL (Structured Query Language) is used to store, retrieve, and manage data in databases.
- It helps arrange data in an organized way, making it easier to analyze and use in AI applications
Key Learning Outcomes
Grasp the Essentials of Databases: Understand how databases function and their interconnected relationships, which are foundational for effective data management.
Proficiency in SQL Commands: Learn to use essential SQL commands like CREATE, READ (SELECT), UPDATE, and DELETE to manage database records efficiently. These CRUD operations are crucial for maintaining clean and updated datasets in AI-driven systems.
Understand Advanced SQL Topics: Develop skills in advanced SQL techniques such as Joins, Subqueries, and Views. Joins allow you to combine data from multiple tables for comprehensive analysis, while subqueries and views help in structuring and preprocessing data efficiently.
Master Stored Procedures: Gain expertise in creating and executing stored procedures for efficient database management, enhancing your capability to handle complex data tasks.
Transaction Management and Table Creations: Acquire knowledge on handling transactions and creating tables and views to ensure data integrity and streamlined processes.
Course Structure
- Lesson 1: Essential SQL Statements
- Lesson 2: Database Backup and Restore Techniques
- Lesson 3: Data Selection and Filtering
- Lesson 4: Data Selection and Ordering
- Lessons 5-16: Advanced SQL Topics, including Window Functions and CTEs
Joins, Subqueries & Views
- Joins – Combine data from multiple tables for comprehensive analysis (INNER, LEFT, RIGHT, FULL JOIN).
- Subqueries – Nested queries used to fetch data within another query.
- Views – Virtual tables storing query results for easy data access and security.
- Used in AI to structure and preprocess data efficiently.
Window Functions & CTEs
- Window Functions – Perform calculations across a set of rows (e.g., ranking, running totals).
- Common Table Expressions (CTEs) – Temporary query results to simplify complex queries.
- Enhances AI-driven analytics by enabling advanced data transformations.
Performance Optimization in SQL
- Techniques like Indexing, Query Optimization, and Partitioning speed up data retrieval.
- Ensures faster AI model training and real-time data analysis.
By incorporating these elements, you’ll build a robust foundation in SQL, equipping you with the skills needed for effective data analysis and management in various applications.
5. Programming for Data Analysis (Python / R)
Introduction to Python/R for Data Analytics
- Python and R are popular programming languages for data analysis, offering powerful tools for data manipulation, visualization, and automation.
- Widely used in AI, machine learning, and big data applications.
Libraries: Pandas, NumPy, Matplotlib, Seaborn (Python) / dplyr, ggplot2 (R)
Python Libraries:
R Libraries:
- Pandas – Handles data manipulation and analysis.
- NumPy – Supports numerical computations and large datasets.
- Matplotlib & Seaborn – Create visualizations like charts and heatmaps.
- dplyr – Streamlines data manipulation with simple functions.
- ggplot2 – Advanced data visualization with customizable graphs.
- These libraries make data analysis faster and more efficient for AI-driven insights.
What are the core components of a Python programming course for Data Analysts?
Core Components of a Python Programming Course for Data Analysts
Python stands out as a versatile tool for data analysts, thanks to its robust capabilities in data manipulation and analysis. If you’re looking into a programming course centered on Python for data analytics, here are the critical components you should expect:
Data Handling and Preprocessing
A crucial aspect is learning how to import, clean, and organize data. Courses typically start with foundational techniques for transforming raw data into a format suitable for analysis.
- Data Importing: Understand how to load data from various sources, including CSV files, databases, and web APIs.
- Data Cleaning: Master techniques to cleanse datasets by removing inconsistencies and handling missing values.
Effective Data Manipulation
Leveraging libraries like NumPy and Pandas, you’ll learn to manipulate data efficiently:
- NumPy: Handle arrays and numerical data for faster computations.
- Pandas: Use this powerful library to manage and analyze structured data seamlessly.
Statistical Analysis and Machine Learning
The course progresses by teaching you to perform statistical analysis and build predictive models:
- Statistical Techniques: Apply statistical methods to analyze data trends and patterns.
- Machine Learning Models: Gain skills in developing models using libraries such as Scikit-learn to turn insights into actionable outcomes.
Data Pipelines and Visualization
Efficiently managing data processes is vital. This involves creating comprehensive data pipelines for seamless analysis and visualization:
- Pipeline Development: Construct automated workflows from data collection to processing.
- Visualization: Use libraries like Matplotlib and Seaborn to create compelling visual representations of your data insights.
Detailed Course Layout
Introduction and Setup: Begin by setting up your Python environment and understanding the basics of programming.
Programming Foundations and Data Techniques: Deep dive into Python syntax and data analytics methodologies. Topics also include programming constructs and essential data operations.
Advanced Topics: Explore data visualization, build intuitive models, and refine data pipelines for more in-depth analysis.
By addressing these areas, a Python programming course for data analysts ensures that learners acquire all the tools needed for effective data manipulation and analysis.
What are the key concepts should a course in R programming covers?
Key Concepts for a Comprehensive R Programming Course
When designing a thorough R programming course, it’s essential to include several core concepts that equip learners with a robust understanding of R’s capabilities.
1. Foundation of R Programming
- Variables and Data Types: Start with the basics by exploring how variables are created and used in R. Understand diverse data types such as numeric, character, and logical.
- Vector Operations: Dive into vectorization, a quintessential feature of R, which allows for efficient data manipulation.
2. Data Structures in R
- Arrays and Lists: Get acquainted with complex data arrangements, leveraging arrays for multi-dimensional data and lists for non-homogeneous collections.
- Data Frames: Master the essential R data frame, a fundamental structure for handling tabular data akin to spreadsheets or SQL tables.
3. Functional Programming and Data Manipulation
- R’s Functional Tools: Explore R’s functional programming elements which offer powerful ways to process data, including using functions like
apply()
,lapply()
, and custom functions. - Data Input/Output: Learn efficient methods for reading data from various sources and writing outputs, ensuring seamless data interchange.
4. Advanced R Techniques
- Statistical Methods: Delve into statistical modeling and analysis which are core strengths of R, covering topics from basic statistics to advanced predictive models.
- Data Visualization: Employ R’s robust visualization capabilities using libraries like ggplot2 to create insightful and dynamic visual representations of data.
Suggested Course Layout
- Introduction and Fundamentals (Lessons 1-5): Build a solid foundation with the basics of R and data handling.
- Advanced Concepts (Lessons 6-10): Progress into complex features like statistical methods and data visualization to deepen proficiency.
This framework ensures that learners walk away with not only the ability to code in R but also the skills to analyze and visualize data effectively.
Data Cleaning & Preprocessing
- Handling missing values, duplicates, and inconsistent data to improve data quality.
- Prepares datasets for AI models, ensuring accuracy and reliability.
Exploratory Data Analysis (EDA)
- Identifies patterns, correlations, and trends in data using statistical summaries and visualizations.
- Helps AI and machine learning models understand data distribution and relationships.
Automating Data Tasks
- Writing scripts to automate repetitive data processing tasks (e.g., data extraction, transformation, and reporting).
- Improves efficiency in AI workflows by reducing manual effort.
6. Data Visualization
1.Principles of Effective Data Visualization
2.Visualization Tools: Power BI, Tableau, Google Data Studio
- Use clear, simple, and meaningful visuals to communicate insights.
- Choose the right chart type (bar, line, pie, heatmap) based on data type.
- Avoid clutter and focus on key takeaways for better decision-making.
- Helps AI-driven systems present complex data in an easy-to-understand format.
- Power BI – Microsoft’s tool for interactive dashboards and real-time reporting.
- Tableau – Advanced data visualization with drag-and-drop features.
- Google Data Studio – Free tool for visualizing data from Google Analytics, Sheets, and more.
- These tools enhance AI-powered data storytelling and business intelligence.
3.Creating Dashboards & Reports
4.Storytelling with Data
- Combine multiple charts and key performance indicators (KPIs) in a single view.
- Provides real-time data insights for businesses and AI-based decision-making.
- Customizable dashboards allow users to interact with and explore data efficiently.
- Turning raw data into a compelling narrative using visuals and insights.
- Helps businesses and AI models communicate trends and predictions effectively.
- Engages stakeholders by making data-driven decisions more understandable.
Advanced Features of Power BI in Data Analytics and Visualization
Power BI offers a robust suite of advanced features that elevate data analysis and visualization to new heights. Here’s a closer look at what’s available:
Interactive Reports and Dashboards
Power BI empowers users to craft dynamic, interactive reports and dashboards. These tools allow for the maintenance and updating of data visuals in real-time, providing an engaging way to explore data insights effectively.Quick Insights and Natural Language Queries
One of the standout features is Quick Insights, which generates automated analytics and discoveries from datasets. Additionally, the Natural Language Query feature allows users to interact with their data conversationally, asking questions in plain English and receiving comprehensive visual responses.Best Practices for Data Layout and Visualization
Power BI emphasizes clarity and effectiveness through recommended practices for data layout and visualization. Designing visuals with intuitive layouts ensures that complex data is presented in an accessible and impactful manner.
What Are the Primary Learning Goals for a Course in Tableau?
Mastery of Visualization Techniques
This course enables you to become proficient in various methods of data visualization. You’ll learn to harness metadata effectively, allowing you to craft diverse and insightful visual representations using Tableau.Development of Interactive Dashboards and Visual Narratives
Acquire the skills necessary to design and modify dynamic dashboards that tell compelling stories through data. This involves constructing visual narratives that captivate your audience, making the data both engaging and accessible.Data Blending and Structured Data Management
Explore advanced techniques such as data blending and creating data extracts. You’ll gain an understanding of how to organize and format data efficiently, ensuring your visualizations are as impactful as possible.
Through these learning objectives, you’ll not only enhance your Tableau skills but also improve your ability to convey complex data in a clear and concise manner.
7. Business Intelligence & Reporting
1.Introduction to Business Intelligence (BI)
2.Understanding KPIs and Metrics
- BI is the process of collecting, analyzing, and visualizing data to support business decisions.
- Uses AI and data analytics to turn raw data into actionable insights.
- Helps organizations track performance, optimize operations, and forecast trends.
- Key Performance Indicators (KPIs) – Measurable values that track business success (e.g., sales growth, customer retention).
- Metrics – Quantitative measurements that support KPIs (e.g., website traffic, conversion rates).
- AI-powered analytics tools help businesses monitor and improve KPIs effectively.
3.Connecting BI Tools to Databases
4.Real-time Data Reporting
- BI tools like Power BI, Tableau, and Google Data Studio connect directly to databases (SQL, NoSQL, cloud storage).
- Ensures real-time data updates for accurate reporting.
- AI-enhanced BI tools automate data extraction and analysis for faster decision-making.
- Provides up-to-the-minute insights using live data from multiple sources.
- Helps businesses react quickly to changes in sales, operations, and customer behavior.
- AI-driven dashboards enhance decision-making with automated alerts and predictive analytics.
8. Advanced Analytics & Machine Learning Basics
Introduction to Predictive Analytics
- Predictive analytics uses historical data and AI techniques to forecast future trends (e.g., customer behavior, sales predictions).
- Helps businesses anticipate challenges, improve strategies, and make data-driven decisions.
Regression & Classification Basics
- Regression – Predicts a continuous value (e.g., predicting sales revenue based on marketing spend).
- Classification – Categorizes data into predefined classes (e.g., spam or not spam emails).
- Both are core techniques in AI and machine learning for making accurate predictions.
Time Series Forecasting
- Analyzes data points collected or recorded at specific time intervals to predict future values (e.g., stock prices, weather forecasting).
- Essential in industries like finance, retail, and energy. AI enhances the accuracy of time series models.
Clustering & Segmentation
- Clustering – Groups similar data points into clusters (e.g., customer segmentation based on purchasing behavior).
- Segmentation – Divides data into subsets to target specific market segments.
- AI and machine learning make clustering more efficient and accurate, leading to better personalization.
Basics of AI in Data Analytics
- AI in data analytics automates decision-making processes using machine learning algorithms.
- It helps identify patterns, predict outcomes, and uncover insights faster than traditional methods.
- AI tools like natural language processing (NLP) and deep learning enhance the depth and accuracy of data analysis.
9. Big Data & Cloud Technologies
Introduction to Big Data & Hadoop
- Big Data refers to massive datasets that are too large to be processed by traditional methods.
- Hadoop is an open-source framework that allows distributed storage and processing of big data across multiple servers.
- Enables businesses to analyze large volumes of data, which is crucial for AI and machine learning models.
Working with Cloud Platforms (AWS, Google Cloud, Azure)
- AWS, Google Cloud, and Azure are cloud platforms that provide scalable storage, computing power, and data analytics tools.
- They help businesses store and process large datasets, enabling real-time insights.
- AI and machine learning models can be deployed and run more efficiently using these cloud services.
Introduction to NoSQL Databases (MongoDB)
- NoSQL databases like MongoDB store unstructured data, allowing for faster and more flexible data retrieval.
- Ideal for big data applications that require flexibility in data modeling and speed.
- Commonly used in AI-driven environments that deal with massive and complex datasets.
Data Warehousing Concepts
- Data Warehousing involves storing data from multiple sources into a single, unified repository for analysis and reporting.
- Helps businesses make data-driven decisions by consolidating structured and unstructured data.
- Supports AI and machine learning by providing a centralized place for data analysis and insights.
10. Data Ethics, Governance & Security
Data Privacy & Compliance (GDPR, HIPAA)
- GDPR (General Data Protection Regulation) and HIPAA (Health Insurance Portability and Accountability Act) are laws that protect personal data and privacy.
- Data analysts must follow these regulations to ensure the ethical handling of sensitive information and avoid legal consequences.
- Compliance is essential in AI and data analytics to maintain trust and data integrity.
Ethical Considerations in Data Analytics
- Data analytics must be conducted with integrity, fairness, and transparency to avoid biases or unethical manipulation of data.
- AI ethics focuses on ensuring algorithms do not discriminate and that data usage is responsible.
- Ensuring ethical decision-making builds trust in AI-driven models and analytics outcomes.
Data Governance Frameworks
- Data Governance involves managing data availability, usability, integrity, and security within an organization.
- Frameworks like DAMA or COBIT ensure that data is used responsibly and consistently across the business.
- Effective data governance is essential for AI projects to maintain high-quality, trusted data.
Data Governance Frameworks
- Cybersecurity focuses on protecting data from unauthorized access, breaches, and cyberattacks.
- Data analysts must implement security measures like encryption, access controls, and secure data storage.
- AI models and analytics rely on secure, accurate data for optimal results, and protecting it is key to maintaining system integrity.
11. Real-World Projects & Case Studies
Hands-on Projects with Real Datasets
- Working with real-world datasets helps develop practical skills in data analysis, from cleaning to visualization.
- Projects simulate actual business challenges, enhancing problem-solving skills and applying AI-powered tools in real scenarios.
Why is a Capstone Project Important in a Data Analyst Syllabus?
A capstone project is a crucial component of a data analyst syllabus for several reasons:
Practical Application of Skills: It enables students to transition from theoretical knowledge to real-world application. By tackling a complex, actual problem, students refine and demonstrate their ability to analyze data, identify trends, and present insights effectively.
Showcasing Expertise: This project serves as a platform for students to exhibit their mastery of data analysis tools and techniques. It’s a robust way to highlight their ability to work with data visualization tools, such as Tableau or Power BI, and apply statistical methods to derive meaningful conclusions.
Problem-Solving Proficiency: Through a capstone project, students develop and showcase their problem-solving skills. They learn to formulate questions, design data-driven solutions, and iterate on their findings, preparing them for the demands of a professional data analytics role.
Portfolio Development: For aspiring data analysts, the capstone project offers a valuable addition to their professional portfolio. Potential employers can review this tangible piece of work, which acts as both a credential and a discussion point during interviews.
Preparation for Real-World Challenges: By engaging in a comprehensive project that simulates industry scenarios, students gain insights into real-world data challenges. They learn to manage data projects end-to-end, from data collection to analysis and reporting, mirroring professional environments and expectations.
In sum, a capstone project not only consolidates learning but also enhances employability by providing a compelling demonstration of skills and expertise in data analysis.
Business Problem-Solving with Data
- Using data analytics to solve real business problems like customer churn, sales forecasting, and market trends.
- AI models and techniques help businesses make data-driven decisions that improve operations and maximize profits.
Industry-Specific Analytics (Finance, Healthcare, Retail, etc.)
- Industry-focused data analytics helps address unique challenges:
- Finance – Risk analysis, fraud detection, and investment predictions.
- Healthcare – Patient care, disease prediction, and treatment optimization.
- Retail – Inventory management, customer segmentation, and personalized marketing.
- These projects provide insights tailored to industry needs, improving AI’s application in each sector.
Resume Building & Portfolio Creation
- Creating a strong data analytics resume and portfolio with real-world projects shows potential employers your practical expertise.
- Including AI-driven projects and case studies helps demonstrate your ability to use advanced tools for solving complex problems.
Are internships included in the course and why are they important?
Internships are generally not a formal requirement in most data analyst courses, but their significance cannot be overstated. While the core curriculum typically focuses on developing essential skills such as data manipulation, statistical analysis, and visualization techniques, the practical application of these skills in a real-world setting is where internships come into play.
Why Internships Matter:
Hands-On Experience: By participating in an internship, students gain invaluable hands-on experience. This exposure helps bridge the gap between theoretical knowledge and practical application.
Industry Insight: Internships offer a unique glimpse into the day-to-day operations of the industry, providing context that enhances classroom learning.
Skill Enhancement: Working on real projects allows students to refine their skills, adapt to new tools, and tackle actual data challenges.
Networking Opportunities: Internships present a chance to build professional relationships with industry experts, which can be crucial for future job placements.
Many educational programs recognize the value of internships and actively assist students in securing opportunities, often collaborating with corporations such as IBM, Google, and Amazon. Although not always part of the syllabus, internships are a vital component of a robust educational experience for aspiring data analysts, significantly boosting their employability and career prospects.
Conclusion of Data Analyst Syllabus
- Comprehensive Learning Path: The syllabus covers everything from data basics to advanced techniques, ensuring a thorough understanding of data analysis.
- Industry-Relevant Skills: Learn popular tools like Excel, SQL, Python, R, and Tableau that are in high demand in the job market.
- Hands-on Experience: Gain practical knowledge through real-world projects and case studies that prepare you for actual job roles.
- Expert Guidance: Get trained by industry experts at DataAnalyticsMasters, who provide personalized support throughout the course.
- Career Opportunities: The course equips you with the skills needed to excel as a data analyst, opening doors to various career opportunities in Hyderabad and beyond.
Recommended blogs :
3.Skills Required For Data Analyst
FAQ'S Of Data Analyst Syllabus
What is the syllabus of data analyst?
The syllabus begins with basic concepts, simple statistics, and Excel, then moves on to advanced topics like Python, R, SQL, and data visualization tools with practical exercises.
What are the 7 steps of data analysis?
Why Data Analytics?
- Identify the Business Problem – Understand the issue or goal that needs data-driven insights.
- Define Data Requirements – Determine what data is needed to solve the problem.
- Collect and Understand Data – Gather relevant data and explore its structure.
- Prepare the Data – Clean and organize data for analysis.
- Visualize the Data – Use charts and graphs to spot patterns.
- Analyze the Data – Apply statistical and analytical techniques to extract insights.
- Deploy the Solution – Implement findings into real-world decision-making.
What are the pre requisites for enrolling Data aanalyst course?
Program Level Required Education Minimum Marks Undergraduate (BCA, BTech CS, etc.) Completed 12th grade 50-60% (may vary) Postgraduate (MS, MTech, MBA) Bachelor’s degree in a related field 60-70% (may vary) When considering a data analyst course, it’s important to recognize not only the academic qualifications but also the necessary foundational skills:
- Basic Math and Statistics: A solid understanding of these subjects is crucial, as data analysis heavily relies on these principles.
- Excel Proficiency: Familiarity with Excel is beneficial, as it is a fundamental tool for data manipulation and visualization.
- Programming Skills: While some courses welcome beginners, others may require knowledge of programming languages like Python or R.
Is data analyst hard?
No, it’s a skill that anyone can learn with time and effort. Many courses, from degree programs to certifications, are designed to teach you the basics and help you apply them in real-world situations.
Is data analyst course easy?
There are plenty of opportunities for aspiring data analysts, but many wonder, “Is data analytics hard to learn?” It’s not the easiest skill, but it’s also not too difficult. With the right guidance and training, anyone can become a skilled data analyst.
Steps to Become a Data Analyst
Learn the Basics – Understand key data analysis skills like statistics, Excel, and SQL. It’s essential to grasp these foundational tools as they form the core of data analysis work.
Get Certified – Complete a data analytics course or degree. Enroll in reputable online courses or academic programs that offer comprehensive training. Look for certifications that include a robust curriculum aligned with industry standards.
Practice Your Skills – Work on real-world data problems. Seek opportunities to apply what you’ve learned through internships, volunteer work, or contributing to open-source projects. This hands-on experience is invaluable for developing practical expertise.
Build a Portfolio – Create projects to showcase your abilities. Document your work on real-world projects and compile them into a portfolio that highlights your analytical skills and problem-solving capabilities. A well-crafted portfolio can be a significant asset when applying for jobs.
Apply for Jobs – Start looking for entry-level data analyst roles. Leverage your connections and professional network to discover job openings. Attend industry events and join online forums to meet others in the field. Platforms like LinkedIn are excellent for connecting with professionals and staying informed about job opportunities.
Is Python mandatory for data analytics?
Knowing Python is very helpful for data analysts. Many employers expect analysts to use Python libraries to make data tasks easier. Learning Python can boost your career opportunities.
Can a 12th pass become data analyst?
The best part is that you can become a data analyst right after the 12th grade. You don’t need a bachelor’s or master’s degree. Just learn the right skills, take relevant courses, and you’re ready to start your career!
Is data analyst a lot of math?
Data analysts should be comfortable with numbers and have a basic understanding of math and statistics. However, most of the work involves following logical steps. You don’t need to be a math expert to succeed in this field.
Is data analyst a safe career?
Data analytics is more than just a trend—it plays a key role in decision-making across industries. With digital growth happening fast, it’s a great career choice both globally and in India.
Recommended Reads for Beginners in Data Analytics
Understanding the Basics – Start with a beginner-friendly book that covers core concepts and essential tools in data analytics.
Lean Six Sigma Approach – Learn how data-driven decision-making enhances quality management and operational efficiency.
Python for Data Analytics – Explore books that teach Python for data analysis, a key skill for aspiring analysts.
Trend Analysis & Future Outlook – Read about industry trends and the evolving role of analytics in the future job market.
DevOps & Data Analytics – Discover how analytics integrates with DevOps to improve software development and certifications.
Future of Data Analytics – Gain insights into emerging technologies, job roles, and the growing impact of analytics.
Can Data analyst syllabus studied online?
Absolutely! The data analyst syllabus can be effectively studied online. Many platforms offer diverse learning experiences tailored for different needs.
Benefits of Online Study
- Flexible Scheduling: Online courses allow you to learn at your own pace, accommodating your schedule.
- Comprehensive Resources: Access a variety of materials, including video lectures, to reinforce learning with visual and auditory elements.
- Interactive Elements: Engage with the course material through quizzes and assignments for hands-on practice.
- Supportive Community: Join forums or discussion groups to connect with peers and instructors, fostering a collaborative learning environment.
What are the typical Duration and Fee Range Data science and Data analytics courses?
Duration and fee of Programs:
Short-Term Courses (3 to 6 months):
Fees: ₹25,000 to ₹75,000
These are typically professional certificate courses, offered by online platforms or institutions.
Post-Graduate/Advanced Diploma Programs (6 to 12 months):
Fees: ₹1,00,000 to ₹4,00,000
These programs provide a more comprehensive understanding of data analytics and may be offered by universities or reputed institutions.
Full-Time Master’s Programs (1 to 2 years):
Fees: ₹4,00,000 to ₹12,00,000
These are typically offered by top universities or B-schools, covering in-depth data analytics along with machine learning, big data, and related subjects.
Why is Python considered as a versatile language for programming and Data analytics?
Python and R are popular for data analysis, offering powerful tools for data manipulation, visualization, and automation.
Why Python?
Versatile & Widely Used: Essential in AI, machine learning, and big data.
Data Handling: Easily imports, cleans, and preprocesses data.
Strong Libraries: NumPy and Pandas simplify data manipulation.
Advanced Analysis: Supports statistical analysis and machine learning.
Integration & Visualization: Builds data pipelines and transforms data into insights.
Python’s flexibility makes it a must-have tool for analyzing, visualizing, and automating data-driven tasks.