AI For Data Analytics
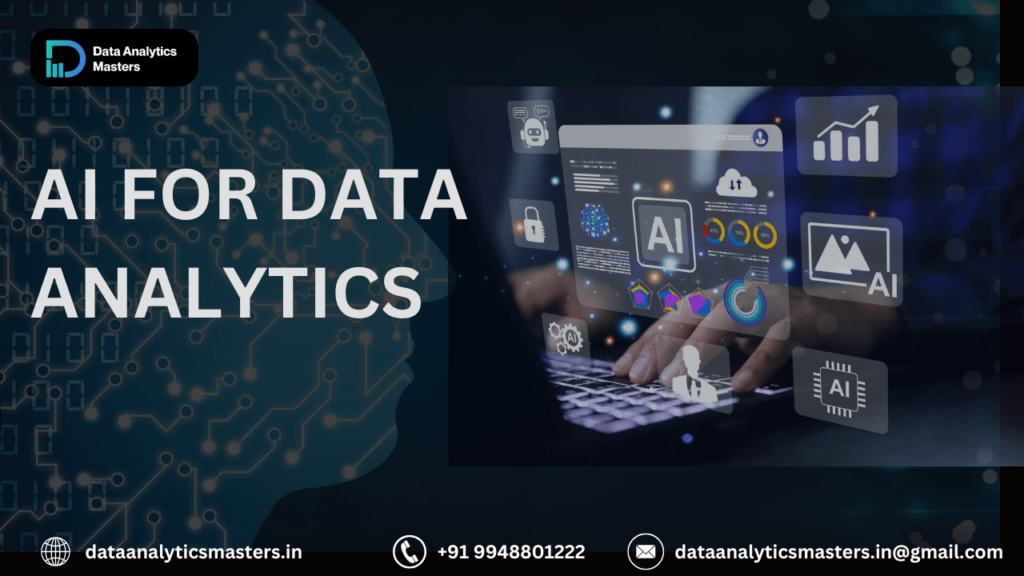
A simple overview of AI for Data Analytics
AI boosts data analytics by automating tasks and improving insights.
It speeds up data processing, detects patterns, and enhances predictions.
Traditional analytics relies on manual work, while AI-driven analytics is faster and more accurate.
AI reduces errors, enables real-time decisions, and improves efficiency.
Challenges include high costs, privacy risks, and the need for AI skills.
AI won’t replace data analysts but will enhance their capabilities.
Learning AI tools and machine learning is key to staying relevant.
The future of AI in data analytics includes automation, self-learning, and smarter insights.
Topics Covered :
1. Introduction to AI for Data Analytics
Overview of AI
- AI (Artificial Intelligence) refers to technologies that allow machines to simulate human intelligence.
- AI systems are capable of performing tasks such as learning, reasoning, and problem-solving, just like humans.
- Over time, AI has evolved and now plays a key role in data analytics by enabling faster, more accurate analysis of large datasets.
Importance of AI in Data Analytics
- Speeds Up Data Processing: AI automates repetitive tasks like data cleaning and analysis, allowing businesses to process data faster.
- Increases Accuracy: AI algorithms improve the accuracy of predictions, reducing human error and uncovering hidden patterns in data.
- Enhances Decision-Making: AI helps businesses make better decisions by providing deeper insights and identifying trends in large datasets.
- Stays Competitive: With AI, companies can analyze data at greater depth and speed, gaining a competitive edge in the market.
2. AI in Data Analytics: How It Works
Data Collection and Preprocessing
- Automates Data Collection: AI can gather data from multiple sources quickly, saving time for analysts.
- Cleansing Data: AI helps remove errors, duplicates, and irrelevant information from datasets, making the data more accurate and ready for analysis.
- Preprocessing: AI can transform raw data into a format that is easier to analyze, such as normalizing values or categorizing information.
Predictive Analytics and Machine Learning
- Machine Learning Models: Machine Learning (ML) is a subset of AI that helps predict future trends based on historical data.
- Making Data-Driven Decisions: AI models can analyze patterns in data and provide insights, helping businesses make better decisions, like forecasting sales or identifying customer behavior.
- Improving Over Time: As more data is collected, ML models improve their accuracy by learning from new data and refining their predictions.
Natural Language Processing (NLP) in Data Analytics
- Analyzing Unstructured Data: NLP helps AI analyze unstructured data, such as customer reviews, social media posts, and voice data.
- Extracting Insights from Text: NLP can identify keywords, sentiment, and trends in text data, providing valuable insights for businesses to understand customer opinions and market sentiment.
- Speech Recognition: NLP can also be used to transcribe and analyze spoken language, making it easier to gather insights from customer service calls or voice-based interactions.
3. How is Data Analytics with and Without AI?
Data Analytics Without AI
- Manual Analysis: Data is analyzed using traditional methods like spreadsheets or basic statistical tools.
- Time-Consuming: The process of manually collecting, cleaning, and analyzing data takes a lot of time.
- Prone to Human Error: Mistakes can happen when handling large amounts of data, affecting the quality of the insights.
- Limited Processing Power: Traditional methods struggle to analyze large datasets, especially in real-time, making it harder to identify trends or patterns.
Data Analytics With AI
- Automated Analysis: AI tools automatically process and analyze data, saving time and improving efficiency.
- Handles Large Datasets: AI can manage and process massive datasets much faster than humans, enabling real-time analysis.
- Uncovers Hidden Patterns: AI identifies patterns and trends that may not be obvious through traditional methods.
- Example: AI can spot anomalies in data or predict future trends, helping businesses make data-driven decisions in real-time and recommending the best actions.
4. Is AI a Threat to Data Analysts?
AI is a Tool, Not a Replacement
- AI Enhances Human Capabilities: AI is designed to augment the work of data analysts, not replace them. It automates repetitive tasks like data cleaning and analysis, allowing analysts to focus on more complex insights.
- AI Assists, Doesn’t Replace Expertise: While AI can handle data processing, it still requires human expertise for interpreting results, making decisions, and providing business context.
AI Will Change Traditional Roles
- Evolving Job Roles: As AI takes over repetitive tasks, the role of data analysts will evolve. They may spend more time on strategic thinking and insight generation, while relying on AI to handle routine processes.
- New Skills Required: Data analysts will need to adapt by learning new AI and machine learning skills to stay relevant in the industry.
How to Survive with AI in Data Analytics
- Reskill and Upskill: Embrace AI tools and learn machine learning, predictive analytics, and AI-based data visualization techniques to stay competitive.
- Focus on Critical Thinking and Problem-Solving: While AI handles data crunching, data analysts must provide business insights, interpret results, and help businesses make decisions based on AI-driven findings.
- AI and Human Collaboration: The future of data analytics lies in the collaboration between AI and human intelligence. Analysts should focus on combining AI’s power with their domain knowledge to derive the most value.
5. Advantages of Integrating AI in Data Analytics
Faster and More Accurate Insights
- Quick Data Processing: AI can analyze large amounts of data much faster than humans, providing real-time insights to decision-makers.
- Improved Accuracy: AI minimizes errors by using algorithms to process data consistently, ensuring more precise insights.
Improved Predictive Capabilities
- Advanced Prediction Models: AI uses machine learning models to predict future trends, customer behaviors, and market shifts more accurately than traditional methods.
- Better Forecasting: Businesses can anticipate changes in the market, customer needs, or financial performance, helping them make informed decisions.
Automation of Repetitive Tasks
- Data Cleaning and Integration: AI automates time-consuming tasks like data cleaning, merging datasets, and organizing information, allowing analysts to focus on more important aspects of analysis.
- Automated Reporting: AI can generate reports automatically, freeing up time for analysts to focus on interpreting the data and providing strategic insights.
Scalability
- Handling Large Datasets: As businesses grow, AI can manage larger datasets and more complex queries without losing performance.
- Adaptable to Growth: AI solutions are flexible and scalable, enabling businesses to handle increasing data volumes as they expand.
6. Disadvantages of AI in Data Analytics
High Initial Investment
- Upfront Costs: Implementing AI solutions requires significant investment in software, hardware, and training for employees.
- Cost of Setup: Small businesses may find it difficult to afford the initial costs of AI technology, making it more suitable for larger companies.
Data Privacy Concerns
- Sensitive Data: AI relies on large volumes of data, including personal and sensitive information, which can raise privacy issues.
- Ensuring Data Security: Companies need to put in place strong privacy protection measures to avoid breaches and protect customer information.
Bias in AI Models
- Bias in Data: AI algorithms may unintentionally learn from biased data, leading to skewed insights or unfair outcomes.
- Monitoring and Adjusting: It’s important to regularly audit AI models and ensure they are not perpetuating existing biases, especially in sensitive areas like hiring or lending.
Dependency on AI Tools
- Over-Reliance on Automation: Heavy reliance on AI for data analysis may cause human analysts to lose critical analytical skills.
- Adaptability Issues: If AI tools fail or malfunction, analysts who depend too much on automation may struggle to handle data without these tools.
7. Future of AI in Data Analytics
Increased Automation and Efficiency
- More Automated Tasks: As AI advances, it will take over even more complex tasks in data analysis, making business operations faster and more efficient.
- Time-Saving: Automation will reduce the need for manual intervention, allowing companies to make data-driven decisions quickly.
Real-Time Decision Making
- Instant Insights: With improvements in AI, businesses will be able to make real-time decisions based on live data, enhancing their agility and responsiveness to changing market conditions.
- Quick Adaptation: AI will enable businesses to react swiftly to customer behavior, market shifts, and other critical factors as they happen.
Integration with Other Technologies
- IoT Integration: AI will work closely with the Internet of Things (IoT), collecting and analyzing data from connected devices to provide more insights.
- Cloud and Blockchain: AI will also integrate with cloud computing for better scalability and blockchain for improved data security and transparency, further enhancing data analytics capabilities.
8. How to Get Started with AI in Data Analytics
Tools and Technologies to Learn
- Python & R: These programming languages are widely used for data analysis and AI, offering many libraries and frameworks for machine learning and data manipulation.
- TensorFlow: An open-source machine learning framework by Google, perfect for building AI models and deep learning applications.
- IBM Watson: A suite of AI tools from IBM that helps businesses use AI for data analysis, including predictive analytics and natural language processing.
Certifications and Courses
- Google AI Certification: A comprehensive program that helps you understand AI concepts and how to apply them in data analytics.
- Microsoft AI Certification: Offers hands-on experience with Azure AI tools, helping you build AI models and analyze data.
- Other Platforms: Explore courses on Coursera, Udemy, and edX to gain both theoretical and practical knowledge in AI and data analytics.
Developing an AI-Driven Data Strategy
- Start Small: Begin by identifying areas of your business where AI can add value, such as automating repetitive tasks or improving decision-making.
- Choose the Right Tools: Select AI tools that align with your business needs, whether it’s for data visualization, predictive analytics, or machine learning.
- Train Your Team: Ensure your team has the necessary training and knowledge to work with AI-powered tools and integrate them into your data strategy.
9. Simple AI Tools for Data Analytics
Beginner-Friendly Tools
Google Colab
- A cloud-based platform that allows users to write and execute Python code in an easy-to-use interface.
- Free to use and perfect for beginners to practice machine learning and data analysis without needing a powerful computer.
- Offers many libraries and resources for data visualization, statistical analysis, and machine learning.
Tableau
- A visual analytics tool that helps you create interactive and shareable dashboards.
- Great for beginners to analyze and visualize data with drag-and-drop features.
- It simplifies complex data analysis and helps businesses make data-driven decisions with visual insights.
Power BI
- A Microsoft tool for business intelligence and data visualization.
- Helps users connect to multiple data sources, clean data, and create interactive reports.
- User-friendly interface that allows you to visualize data trends and generate insights without complex coding.
No-Code AI Tools
DataRobot
- A no-code AI platform designed to make machine learning accessible to everyone, even without programming experience.
- You can easily upload datasets, and DataRobot will automatically build, train, and evaluate models.
- It’s great for automating predictive analytics and identifying trends from your data with minimal effort.
BigML
- A no-code machine learning tool that provides a simple interface for building predictive models.
- Allows users to upload data and quickly create models for tasks like classification, regression, and clustering.
- Ideal for those looking to integrate AI into their workflows without needing coding skills.
10. The Role of AI in Data-Driven Decision Making
Impact on Business Decisions
Uncover Hidden Trends
- AI analyzes large datasets to reveal hidden patterns, trends, and correlations that would be hard to spot manually.
- This helps businesses make more informed decisions based on data-driven insights rather than relying on gut feelings or intuition.
Improved Accuracy
- AI models predict future trends with higher accuracy, ensuring better decision-making in areas like sales, marketing, and customer service.
- With machine learning, AI continuously learns and improves, adapting to new data for more precise decisions.
Faster Decision-Making
- AI can process data and provide insights faster than traditional methods, allowing businesses to make real-time decisions and respond quickly to market changes.
Optimized Strategy
- AI helps businesses optimize marketing strategies, product pricing, and resource allocation, leading to more effective use of time, money, and resources.
Examples of AI in Action
Marketing
Personalized Recommendations
- AI analyzes customer data to provide personalized product recommendations based on individual behavior, preferences, and past purchases.
- For example, Amazon and Netflix use AI to suggest products and movies, increasing customer satisfaction and sales.
Finance
Fraud Detection
- AI can analyze transaction data in real-time to detect fraudulent activities or unusual behavior.
- Banks and credit card companies use AI to flag transactions that don’t match typical patterns, helping prevent fraud.
Customer Service
Chatbots and Virtual Assistants
11. Basic AI Skills You Need for Data Analytics
Foundational Knowledge
Statistics
- Understanding basic statistics (mean, median, standard deviation) is crucial for analyzing and interpreting data.
- Statistics helps you make sense of data distributions and draw conclusions from patterns in datasets.
Data Visualization
- Learn how to represent data visually through charts, graphs, and dashboards to make it easier to interpret and present findings.
- Tools like Tableau and Power BI help create interactive and insightful visualizations.
Programming (Python or R)
- Python is widely used in AI and data analytics due to its simplicity and extensive libraries like pandas, numpy, and matplotlib.
- R is another popular programming language specifically designed for statistical computing and data analysis, great for beginners in analytics.
Learning Path
Step 1: Learn the Basics of Data Analytics
- Start with an understanding of data collection, cleaning, and basic statistical analysis.
- Practice with small datasets to familiarize yourself with tools like Excel or Google Sheets.
Step 2: Learn Data Preprocessing
- Understand how to clean and prepare data by handling missing values, normalizing data, and converting data types.
- This step is crucial for ensuring that the data is ready for analysis and machine learning.
Step 3: Learn Data Analysis Techniques
- Dive deeper into exploratory data analysis (EDA) to identify trends, correlations, and patterns.
- Learn about regression analysis, classification, and clustering techniques, which are essential for machine learning.
Step 4: Learn Machine Learning Basics
- Begin with supervised learning (regression and classification) and unsupervised learning (clustering).
- Use Python libraries like scikit-learn to build your first machine learning models.
Step 5: Advance to Deep Learning
- After getting comfortable with basic machine learning, explore more complex models like neural networks and deep learning using tools like TensorFlow or Keras.
Conclusion
Recap the Importance of AI in Data Analytics
- Transforming the Landscape: AI is revolutionizing the field of data analytics by providing faster, more accurate, and deeper insights that help businesses make informed decisions quickly.
- Driving Efficiency: With AI, businesses can automate tasks, process large datasets, and uncover patterns that would be hard to detect manually.
Encourage Continued Learning
- Stay Ahead of the Curve: The world of AI and data analytics is evolving rapidly. To stay competitive, it’s important to continue learning and mastering new AI technologies and tools.
- Integrate AI into Processes: As you gain new skills, integrate AI into your data analytics processes to improve decision-making and operational efficiency.
AI and Human Collaboration
- Working Together for Success: While AI handles data processing and analysis, human expertise is crucial for interpreting results and making strategic decisions.
- Maximize Potential: Combining AI’s power with human insight will lead to the best outcomes in data analytics, allowing businesses to thrive in an increasingly data-driven world.
Additional Resources:
FAQ'S Of AI For Data Analytics
Can AI be used for data analysis?
Data analysts in various industries can use AI to improve their work. AI can help in areas like detecting credit card fraud in real-time, supporting disease diagnosis, predicting demand in retail, and analyzing player behavior in gaming apps. It can be useful for many different industry needs.
How to learn AI for data analytics?
Key skills:
- Good knowledge of Python, R, and SQL.
- Understanding of Machine Learning and AI.
- Ability to analyze data using statistics, numbers, and predictions.
- Skills in creating charts and reports to show data insights.
- Strong communication and presentation abilities.
What is data analytics in artificial intelligence?
Data analytics is the process of turning raw data into valuable information. This project aims to create systems that use new technology like artificial intelligence and machine learning to make the data analysis process automatic.
Will AI replace data analysts?
Why AI Won’t Replace Data Analysts
- Human skills: Data analysts use creativity, critical thinking, and understand context.
- Human interaction: Analysts build trust through learning, adapting, and apologizing.
- Business knowledge: Analysts understand the business and how data decisions affect it.
- Ethics: Analysts ensure data is used ethically and follows regulations.
How AI Can Help Data Analysts
- AI can automate tasks like data collection and analysis.
- AI can assist with data visualization and creating interactive dashboards.
- AI can support analytics and coding tasks.
How to Prepare for the Future
- Learn skills in data mining, machine learning, and programming (Python, R).
- Take online courses to stay updated on new methods in data analysis and AI.
What is the salary of an AI data analyst?
The salary of a Data Analyst at AI Variant ranges from ₹1 lakh to ₹7 lakhs per year, depending on experience (from less than 1 year to 5 years). These estimates are based on the salaries of 272 employees at AI Variant.
How to become an AI data analyst?
- Start with basic education.
- Improve your technical skills.
- Work on projects using real data.
- Create a portfolio to showcase your work.
- Practice presenting your results.
- Apply for an entry-level data analyst job.
- Think about getting certifications or an advanced degree.
Can ChatGPT replace a data analyst?
ChatGPT and similar models are not meant to replace data analysts. While they can help with tasks related to understanding language, they don’t have the specific skills, knowledge, and analysis abilities that human data analysts have.
Is there a future in data analytics?
Why Data Analytics is a Good Career
- Data-driven decisions are becoming more important in many industries.
- Companies rely on data to improve customer experience, optimize supply chains, and boost sales.
- Data analysts play a key role in business decision-making.
How the Field is Evolving
- AI and machine learning are automating complex tasks.
- Data storytelling and visualization help turn data into valuable insights.
- Edge computing brings data analysis closer to the source.
How to Future-Proof Your Career
- Learn to use AI tools.
- Take comprehensive training programs.
- Focus on continuous learning.
- Develop skills like critical thinking and problem-solving.
In-Demand Skills
- SQL, Python, and R
- Data visualization tools like Power BI and Tableau
- MS Office, especially Excel
- A degree in statistics, computer science, or math
Data Analytics Masters is a top institute in Hyderabad offering expert-led data analytics course in Hyderabad with hands-on training in Python, SQL, Power BI, and Machine Learning to boost your career.