Data Collection Methods And Techniques
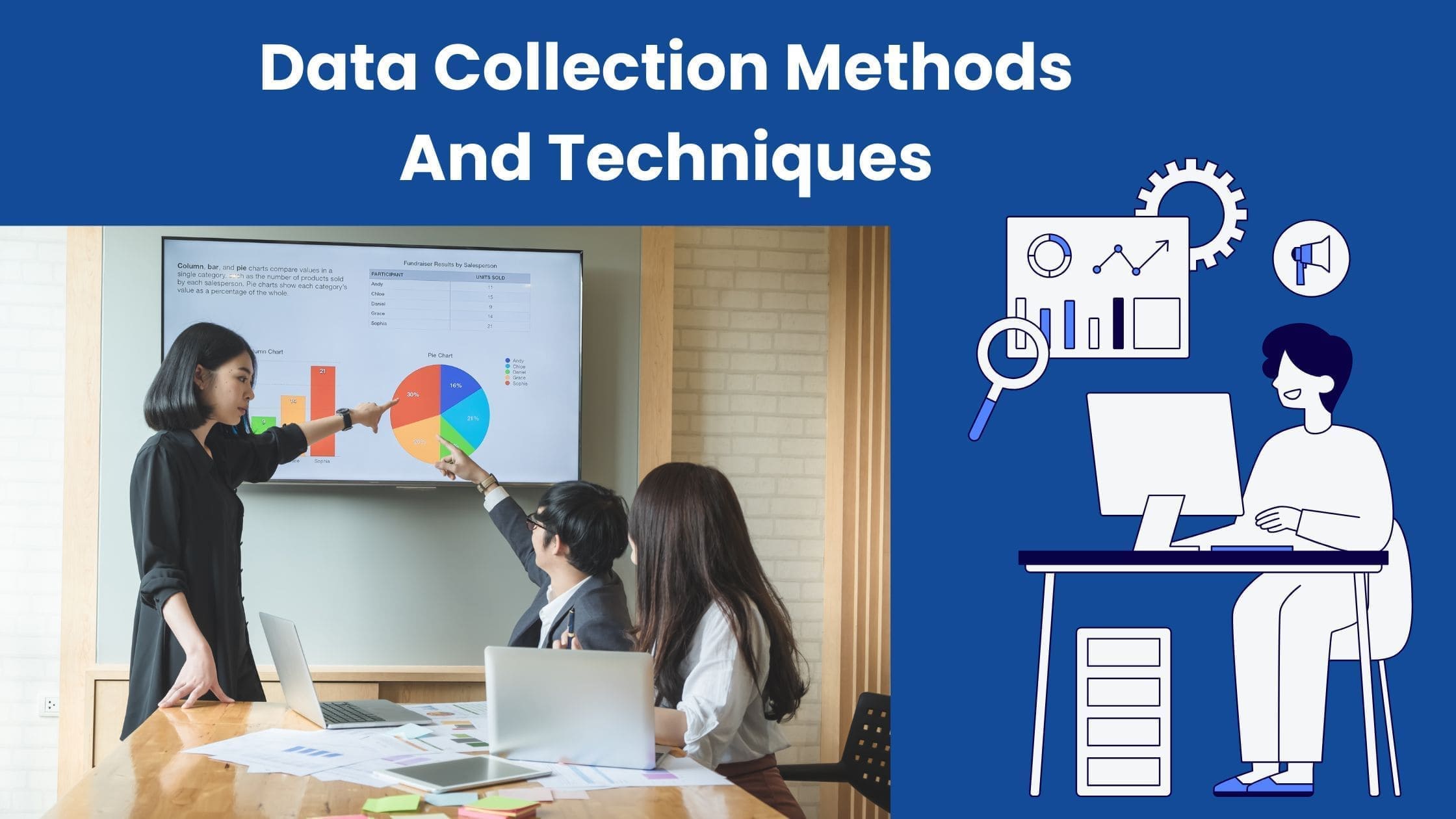
What is Data Collection?
Introduction Section
Data collection is the systematic process of gathering information from various sources to get a clear picture of a topic, phenomenon, or situation. It’s a foundational step in research, analysis, and decision-making across industries—whether in business, healthcare, education, or social sciences.
At its core, data collection helps researchers and organizations gather facts and figures in a structured way. These facts could come from surveys, interviews, online forms, observations, or even past records. Depending on the goals, the data may be numerical (quantitative) or descriptive (qualitative).
In simple terms, data collection answers questions like:
- What is happening?
- How often is it happening?
- Why is it happening?
Without proper data collection, any analysis or decision risks being inaccurate or incomplete.
Why Do We Need Data Collection?
We need data collection because it supports evidence-based decision-making. Whether you’re a company looking to improve a product, a teacher tracking student performance, or a policymaker planning a new initiative—data provides the factual foundation.
Here are some key reasons why data collection is essential:
- To identify problems: Collecting data helps spot trends, issues, or inefficiencies early on.
- To make informed decisions: Rather than guessing, leaders can rely on real insights.
- To measure impact: Data helps assess whether actions or strategies are effective.
- To improve processes: It guides refinement and innovation.
- To meet goals: Tracking key metrics ensures goals are being met or adjusted appropriately.
Simply put, no effective strategy can be built without reliable data.
What are Data Collection Methods?
Data collection methods are the techniques or tools used to gather data from various sources. They determine how the data is collected—whether by asking people directly (like surveys or interviews), observing behavior (like fieldwork), or reviewing existing records and databases.
These methods are broadly categorized into:
- Primary data collection methods (data you gather yourself)
- Secondary data collection methods (data collected by others)
Each method is chosen based on the type of information needed, the research goal, the target audience, and available resources. Selecting the right method is crucial—it affects the accuracy, cost, and usefulness of the data.
Importance of Data Collection Methods And Techniques
The method you choose to collect data can make or break your entire research or project. Well-planned data collection methods ensure:
- Accuracy: You get reliable and valid information.
- Consistency: Data is gathered in a standardized way, making it easy to compare.
- Efficiency: Time and resources are used wisely.
- Relevance: Only the most important and useful data is captured.
For example, using a mobile-based survey tool in a rural area where internet access is limited may lead to incomplete data. Similarly, relying on outdated secondary data might skew your results. That’s why choosing the right data collection method is not just a technical step—it’s a strategic decision.
Data Collection Methods Types & Examples
Types of Data Collection Methods
Data collection methods can be broadly categorized into primary and secondary methods. Each type has its own techniques, advantages, and use cases.
1. Primary Data Collection Methods
Primary data collection involves gathering new data directly from original sources. This data is specific to the purpose at hand and is collected for the first time. It is typically more accurate and relevant but also time-consuming and resource-intensive.: Quantitative Methods
These methods involve collecting numerical data that can be measured, counted, and statistically analyzed. The goal is to quantify opinions, behaviors, or other defined variables.
Surveys and Questionnaires
Surveys are one of the most widely used tools in quantitative data collection. They consist of structured questions with predefined response options. They can be distributed via paper, phone, email, or online platforms like Google Forms or SurveyMonkey.
Advantages:
- Easy to distribute to a large audience
- Standardized responses for analysis
- Cost-effective for large samples
Example: A company sends out a customer satisfaction survey to collect feedback on a recent product launch.
Interviews
In quantitative interviews, researchers ask a fixed set of questions in a structured format, often using yes/no or multiple-choice responses. These are useful when personal interaction is needed, but the data still needs to be standardized.
Advantages:
- Clarification of responses
- Higher response accuracy
- Can be conducted face-to-face or via phone/video
Example: A market research analyst interviews 100 shoppers using the same questionnaire to understand buying preferences.
Qualitative Methods
Qualitative data collection focuses on understanding meanings, experiences, and opinions. This method captures detailed, descriptive information rather than numbers.
Observations
This involves watching and recording behaviors or events in their natural setting without influencing them. It’s useful for collecting real-time, unbiased information.
Advantages:
- Direct insight into real-world behavior
- No reliance on respondent memory or honesty
- Ideal for studies involving human behavior or interaction
Example: A teacher observes classroom participation to understand student engagement.
Focus Groups
A focus group is a guided discussion involving a small group of people on a particular topic. A moderator facilitates the session to gather diverse opinions and insights.
Advantages:
- Rich, detailed data
- Helps uncover ideas or issues not captured by surveys
- Allows observation of group dynamics
Example: A tech company hosts a focus group to test user reactions to a new app interface.
Forms
Forms are a blend of quantitative and qualitative tools. They allow structured data entry while offering optional fields for comments or suggestions. Forms are widely used in healthcare, education, and HR settings.
Advantages:
- Organized and easy to analyze
- Can collect large volumes of data quickly
- Useful for standardized reporting
Example: Patients fill out a medical history form before their appointment.
2. Secondary Data Collection Methods
Secondary data collection uses existing data that has already been collected by someone else, often for different purposes. This includes data from books, websites, reports, databases, government records, and academic journals.
Advantages:
- Time-saving and cost-effective
- Often easily accessible
- Useful for historical or trend analysis
Examples:
- A researcher using World Bank statistics for economic analysis
- A student referring to census data for a project
- A company analyzing competitor data from public reports
Secondary methods are best when foundational knowledge is needed or when primary data is difficult to gather. However, researchers must assess the credibility, relevance, and accuracy of secondary sources before using them.
How to Collect Data Getting Started
Effective data collection starts with a solid plan. Before gathering data, it’s important to clearly define what you need, where you’ll get it from, and how you’ll ensure the quality and accuracy of the information. Below are the essential steps for successful data collection.
1. Define Your Goals
The first step in any data collection process is to define clear and specific goals. What do you want to learn or understand? Are you trying to measure customer satisfaction, track employee performance, or study a social trend?
Why this matters:
Having well-defined objectives helps you stay focused and ensures you collect only the data that is relevant and useful.
Example:
If your goal is to understand customer preferences, your data should focus on buying habits, feedback, and usage patterns.
2. Identify Your Data Sources
Once you know your goals, you need to identify the best sources to gather the required data. These could be people (e.g., customers or employees), systems (e.g., CRM or ERP), or documents (e.g., reports, articles, databases).
Types of sources:
- Primary sources: Direct interactions (e.g., surveys, interviews)
- Secondary sources: Existing records or data (e.g., published research, government data)
Example:
For studying employee performance, your sources might include HR databases, supervisor feedback, and self-evaluations.
3. Choose Your Data Collection Method
Your choice of method depends on your objectives, available resources, and type of data (qualitative or quantitative). Methods can range from surveys and interviews to observation or form analysis.
Key considerations:
- Do you need numerical or descriptive data?
- Is in-person or remote data collection more suitable?
- What level of detail do you require?
Example:
A startup may choose online surveys to gather user feedback quickly and at scale.
4. Determine Your Sampling Method
If you’re not collecting data from the entire population, you’ll need a sampling strategy. A sample should be representative of the larger group to ensure your results are valid.
Common sampling techniques:
- Random sampling: Every subject has an equal chance of being selected
- Stratified sampling: The population is divided into subgroups and samples are drawn from each
- Convenience sampling: Subjects are selected based on availability
Example:
A researcher may randomly select 100 students from different schools to study academic performance.
5. Identify and Train Data Collectors
If others are collecting the data for you (e.g., survey administrators or observers), it’s essential they are properly trained. Consistent data collection ensures accuracy and reduces bias.
Training should cover:
- How to ask questions or record observations
- How to use tools or software
- How to handle respondent concerns or ethical issues
Example:
Before launching a phone survey, the research team trains its staff on how to ask questions neutrally and log responses correctly.
Steps in the Data Collection Process
Once the planning is complete, it’s time to put your data collection strategy into action. This phase involves designing effective tools, gathering data efficiently, and ensuring the data is clean and usable. Proper execution ensures the insights you gain are valid and reliable.
6. Design and Test Your Instruments
Your “instruments” are the tools or methods you use to collect data — like surveys, interview guides, observation checklists, or web forms. Designing these tools carefully is critical to collecting accurate and meaningful data.
Perform Cognitive Interviewing
Cognitive interviewing involves testing your questions or forms on a small group of people before full rollout. This helps you identify confusing or misleading items and refine them.
Example:
If a survey question asks, “Do you frequently shop online?”, test whether people interpret “frequently” the same way. Responses may vary unless you define it (e.g., “more than once a week”).
Put Your Instrument to the Test
Conduct a pilot test or trial run of your instrument with a small group. This helps you check timing, understand how respondents react, and find technical or content-related issues before full-scale deployment.
Tip:
Analyze the pilot results — are you getting the data you need? Is anything missing or redundant?
7. Collect Your Data
Now it’s time to implement your data collection. Ensure that your team follows standardized procedures so the data remains consistent and unbiased across all sources.
Tips for effective data collection:
- Follow ethical guidelines, including consent and privacy.
- Use automation where possible (e.g., digital forms or sensors).
- Monitor progress in real-time if tools allow it.
Example:
A company uses Google Forms to collect customer feedback, ensuring every form is time-stamped and verified automatically.
8. Clean and Organize Your Data
Raw data often contains errors, duplicates, or missing values. Cleaning and organizing it is essential before analysis.
Steps in data cleaning:
- Remove duplicates
- Fix or remove inaccurate entries
- Fill in or flag missing values
- Convert formats (e.g., date formats, text to numbers)
Why this matters:
Clean data increases the accuracy of your results and makes it easier to analyze.
Example:
If a spreadsheet has multiple spellings of a product name, standardizing them ensures accurate reporting in pivot tables or dashboards.
What Happens After Data Collection?
Collecting data is only the beginning. Once you’ve gathered information, the next step is to make sense of it, derive conclusions, and ensure it is securely stored. This stage transforms raw data into valuable insights that can drive decisions, strategies, or innovations
Process and Analyze Your Data
Processing data means converting raw information into a structured format that’s ready for analysis. This includes cleaning, categorizing, and formatting the data.
Steps in processing and analyzing:
- Clean: Remove duplicates, fix errors, standardize formats.
- Organize: Use tools like spreadsheets, SQL, or data software to arrange data in tables or dashboards.
- Analyze: Use statistical methods or software like Excel, Python, R, or SPSS to identify patterns, trends, or relationships.
Example:
If you conducted a customer satisfaction survey, you might calculate the average score for each question and create visual charts to identify common feedback themes.
Interpret and Report Your Results
Interpreting data means understanding what the numbers or observations tell you about your research question. It’s about translating analysis into insights.
How to interpret and report effectively:
- Connect results to your original goals or hypotheses.
- Use visual aids like graphs or charts to communicate findings clearly.
- Keep reports focused, actionable, and tailored to your audience (e.g., executives, clients, students).
Example:
A business might find that users aged 18–24 prefer mobile-friendly websites. The insight? Prioritize mobile design in future updates.
Safely Store and Handle Data
Data, especially when sensitive or personal, must be stored and handled securely to comply with laws (like GDPR) and protect privacy.
Best practices for data storage:
- Use password-protected databases or cloud storage.
- Limit access only to authorized personnel.
- Back up data regularly.
- Anonymize or encrypt data when necessary.
Example:
A university research team stores survey responses in a secured cloud system with encryption and restricts access to only lead researchers.
Common Data Collection Challenges
Collecting data can be highly rewarding, but it’s not without its challenges. From ensuring accuracy to protecting sensitive information, researchers and organizations must navigate various obstacles. Recognizing these challenges early helps in developing better strategies to overcome them.
Data Quality and Consistency
Problem: Inaccurate, incomplete, or inconsistent data can ruin your analysis. It may come from poorly designed surveys, untrained data collectors, or respondents misunderstanding questions.
Why it matters: Low-quality data leads to unreliable conclusions, which can negatively affect business or research outcomes.
Solution:
- Pre-test your data collection tools.
- Use standardized formats and clear instructions.
- Train all team members on how to gather and record data consistently.
Example: If one team member records temperature in Celsius and another in Fahrenheit, the dataset becomes misleading unless standardized.
Data Security
Problem: With increasing regulations and awareness around data privacy, protecting collected data is a legal and ethical obligation. Leaks or breaches can result in serious consequences.
Why it matters: Personal, health, or financial data must be secured to protect participants and maintain trust.
Solution:
- Use secure tools for data collection and storage.
- Encrypt sensitive data and limit access.
- Stay compliant with laws like GDPR or HIPAA.
Example: If you’re collecting employee feedback, use secure survey tools and ensure anonymity to protect identities.
Offline Data Collection
Problem: In remote areas or fieldwork settings, internet connectivity may be unavailable, making online tools impractical.
Why it matters: A lack of internet access can disrupt the data collection timeline and reduce efficiency.
Solution:
- Use mobile apps or tools that work offline and sync data when reconnected.
- Prepare backup paper forms if necessary.
Example: Tools like KoboToolbox or SurveyCTO allow data collection without internet, ideal for rural health studies or disaster relief efforts.
Issues Related to Maintaining the Integrity of Data Collection
Problem: Bias, data manipulation, or changes in data collection procedures can impact the integrity of the entire process.
Why it matters: If the process lacks transparency or uniformity, your findings may be questioned or invalidated.
Solution:
- Create a clear data collection protocol and stick to it.
- Document every step for reproducibility.
- Audit samples of data regularly for consistency.
Example: If a researcher changes interview questions mid-way, it may skew the results. A protocol helps avoid such inconsistencies.
Utilizing Technology to Collect Data
Today’s data collection has evolved far beyond paper forms and clipboards. With the help of technology, organizations can gather, manage, and analyze large volumes of data with higher accuracy and efficiency. Below are essential tools grouped by purpose to guide you in choosing the right tech for your data collection process.
Data Collection Tools for Entry, Management, and More
These tools allow users to design forms, gather responses, and organize data into structured formats.
Popular Tools:
- Google Forms / Microsoft Forms: Simple, cloud-based tools for quick surveys.
- Airtable: Combines spreadsheet features with database capabilities.
- Zoho Forms: Offers advanced logic, workflows, and integrations for larger teams.
Best For: Basic to intermediate data collection and internal team collaboration.
Data Analysis, Visualization, Reporting, & Workflow Tools
Once data is collected, it must be analyzed and visualized to derive insights. Tools in this category also support reporting and automation.
Popular Tools:
- Microsoft Power BI / Tableau: Great for creating dashboards and interactive visualizations.
- Google Data Studio: Integrates easily with Google products for real-time reporting.
- KNIME / RapidMiner: Workflow automation and data modeling capabilities.
Best For: Researchers, analysts, and teams seeking real-time insights and automated reports.
Survey Tools
These platforms are optimized specifically for surveys, supporting question branching, logic, and analytics.
Popular Tools:
- SurveyMonkey / Typeform: Customizable surveys with logic jumps.
- Qualtrics: Enterprise-grade with deep analytical capabilities.
- LimeSurvey: Open-source and flexible, ideal for academic research.
Best For: Market research, academic studies, customer feedback.
Interview and Focus Group Tools
For collecting qualitative data through spoken interactions, these tools assist in recording, transcribing, and organizing insights.
Popular Tools:
- Zoom / Microsoft Teams: Virtual interviews and focus groups with recording features.
- Otter.ai / Descript: Automatic transcription and audio editing.
- Nvivo: Used to code and analyze text from interviews and discussions.
Best For: Social science research, UX research, team discussions.
Observation and Field Data Collection Tools
These tools allow real-time data entry in fieldwork settings, especially when observing behaviors or environmental factors.
Popular Tools:
- Fulcrum: Great for structured observation with offline support.
- Open Data Kit (ODK): Open-source, customizable forms for mobile data gathering.
- Fieldscope: Designed for citizen science and community-based research.
Best For: Field researchers, environmental studies, public health teams.
Mobile Data Collection Tools
When collecting data on the go, mobile apps are essential for efficiency and accuracy.
Popular Tools:
- KoboToolbox: Ideal for humanitarian and field data collection, even offline.
- SurveyCTO: Secure mobile surveys with powerful logic and encryption.
- Magpi: Mobile-friendly, with media upload support and GPS tagging.
Best For: NGOs, field surveys, emergency response teams.
Qualitative Data Analysis Tools
For analyzing unstructured data such as open-ended responses, transcripts, and text files, these tools assist in identifying patterns and themes.
Popular Tools:
- Nvivo / MAXQDA: Widely used for coding qualitative data.
- ATLAS.ti: Great for grounded theory research and content analysis.
- Dedoose: Web-based and suitable for mixed-methods research.
Best For: Researchers handling interview transcripts, feedback, or open comments.
General Data Collection and Management Tools
These tools offer end-to-end solutions for everything from form creation to storage and analysis.
Popular Tools:
- REDCap: Used in medical and academic research with strong data security.
- Qualtrics XM Platform: A comprehensive solution for enterprise data management.
- Google Workspace / Microsoft 365: Integration-friendly tools for small teams.
Best For: Organizations needing all-in-one solutions for research or program tracking.
Master Your Data Collection Process With SurveyCTO
SurveyCTO is a secure, scalable platform specifically designed for high-quality data collection in various settings, from field research to enterprise surveys. Its core strength lies in its ability to maintain data integrity even in low-connectivity environments.
Key Features of SurveyCTO:
- Offline Data Collection: Ideal for remote areas. Users can collect data without internet access and sync when back online.
- Advanced Form Design: Offers support for complex skip logic, validations, multimedia inputs, and calculated fields.
- Data Encryption: End-to-end encryption ensures data security from collection to storage.
- Real-Time Monitoring: Supervisors can monitor data collectors’ performance and get alerts for quality issues.
- Integration: SurveyCTO integrates smoothly with Excel, Stata, R, and other analysis tools, streamlining workflows.
Use Cases:
- International development projects
- Public health surveys
- Academic research and longitudinal studies
- Large-scale monitoring and evaluation programs
Why Choose SurveyCTO?
If your data collection process involves challenging field conditions, multiple data collectors, or requires secure handling of sensitive information, SurveyCTO ensures accuracy, compliance, and reliability at scale.
How Can QuestionPro Help to Create Effective Data Collection?
QuestionPro is a powerful and intuitive online survey platform designed to simplify the data collection process across industries. It helps users create detailed, logic-based surveys while offering insightful analytics to turn responses into actionable data.
Core Advantages of Using QuestionPro:
- User-Friendly Interface: Drag-and-drop question types make survey creation fast, even for beginners.
- Advanced Logic and Branching: Build personalized survey flows with skip logic, display logic, and piping.
- Multi-Channel Distribution: Send surveys via email, SMS, QR codes, or social media platforms.
- Real-Time Analytics Dashboard: View charts, trends, and filters instantly as responses roll in.
- Panel Integration: Access ready-made respondent panels for targeted data collection campaigns.
Ideal For:
- Customer satisfaction surveys
- Market research
- HR and employee feedback
- Product feedback and academic studies
Why Use QuestionPro?
If you need a fast, flexible solution for digital surveys with built-in analytics and diverse deployment options, QuestionPro offers a comprehensive ecosystem that empowers both novice users and experienced researchers.
Moving Ahead Iterative Steps in Data Collection
Data collection is rarely a one-time event—it’s an iterative process that demands thoughtful planning and regular refinement. As your research or business needs evolve, so should your data collection strategy. Implementing best practices helps ensure accuracy, efficiency, and value in every data point collected.
Data Collection Considerations and Best Practices
1. Take Into Account the Price of Each Extra Data Point
Every additional data point can increase the time, cost, and complexity of your project. Ask yourself: Is this data essential to the objectives? Unnecessary data can lead to information overload and delay analysis. Focus on quality over quantity to ensure resources are well spent.
2. Plan How to Gather Each Data Piece
Decide the most efficient and accurate method for collecting each piece of information—surveys, observations, sensor inputs, interviews, etc. Tailoring the data collection method to each type of data improves overall quality and reduces risk of errors or missing data.
3. Think About Mobile Device Usage
With fieldwork and remote data gathering becoming more common, mobile data collection offers real-time inputs, offline capabilities, and user-friendly interfaces. Ensure your tools and forms are optimized for smartphones and tablets to maximize flexibility and responsiveness.
4. Carefully Consider the Data You Need
Before launching any survey or form, define what information is mission-critical. Identify core variables and avoid “nice to have” questions that add little analytical value. This ensures your dataset remains focused, lean, and relevant.
5. Consider Identifiers
Data is most valuable when it can be linked back to specific entities—individuals, households, businesses, or locations. Use unique identifiers (IDs, phone numbers, geo-tags) responsibly to enable cross-referencing, while respecting privacy and ethical standards.
6. Mobile Data Collection is the Way to Go
From GPS tagging to real-time updates, mobile devices empower modern data collectors. They reduce human error, eliminate paperwork, and allow instant uploads. For dynamic and distributed teams, mobile-first strategies are often the most scalable and reliable option.
Career Aligned Learning Paths
If you’re interested in harnessing the power of data to solve real-world problems, there are a variety of learning paths designed to align with your career goals. Whether you’re an aspiring data scientist or a business analyst eager to explore the frontier of Generative AI, these programs are tailored to equip you with job-ready skills.
Become a Data Science & Business Analytics Professional
Build a strong foundation in data science and analytics while also embracing the future of AI-driven decision-making. These programs offer practical, in-demand training from top universities and industry experts.
Data Scientist
Start with the essentials—statistics, Python programming, machine learning, and data visualization. The data scientist role is ideal for professionals who love solving complex problems using data and algorithms.
Professional Certificate in Data Science and Generative AI
This course merges the foundations of data science with the exciting innovations of Generative AI. Learn to design intelligent systems, work with large datasets, and use models like GPT for practical use cases.
Advanced Executive Program In Applied Generative AI
Geared toward managers and senior professionals, this program emphasizes strategic application of Generative AI in business functions such as marketing, operations, and finance.
Professional Certificate Program in Generative AI and Machine Learning – IITG
An advanced program from the Indian Institute of Technology Guwahati, combining rigorous ML theory with real-world Generative AI tools and practices.
Generative AI for Business Transformation
Designed for business professionals, this course helps you understand how Generative AI can reshape operations, automate tasks, and improve customer experience through smart implementation.
Applied Generative AI Specialization
This specialization is focused on real-world applications of Generative AI. Build projects, understand model deployment, and become proficient in AI integration into products.
Get Affiliated Certifications with Live Class Programs
In addition to structured self-paced courses, many learners benefit from live class programs that offer peer interaction, expert mentorship, and real-time project work. Here’s what past learners have to say:
Here’s what learners are saying:
A. Anthony Davis
“The live sessions made complex AI concepts incredibly approachable. I loved the hands-on labs and real-time project feedback from industry experts.”
Magdalena Szarafin – Manager, Group Accounting & Data Analytics, Chemicals
“I gained deep insights into how AI tools can transform financial reporting and forecasting. The practical examples and faculty experience made a huge impact on my role.”
Conclusion
Key Takeaways from This Guide
- Data collection is foundational to making informed, evidence-based decisions across industries—from healthcare and education to marketing and technology.
- There are two main types of data collection: primary methods (such as surveys, interviews, and observations) and secondary methods (such as reports, databases, and existing research).
- A successful data collection process involves clear goal setting, choosing the right tools and methods, training data collectors, and implementing a structured plan for gathering, cleaning, and analyzing data.
- Technology plays a critical role in streamlining data collection—using tools like SurveyCTO, QuestionPro, mobile data apps, and visualization software improves accuracy and efficiency.
- Post-collection steps such as data processing, interpretation, reporting, and secure storage are just as important as the initial collection phase.
- Being aware of common challenges (e.g., data security, offline access, consistency issues) and following best practices helps ensure data integrity and usability.
- Career pathways in data science, analytics, and AI increasingly depend on a strong understanding of data collection methods and tools.
Whether you’re conducting field research, running a business survey, or developing a new app, mastering data collection is a critical step in turning raw information into actionable insight.
FAQ s
1. What is data collection with example?
Data collection is the process of gathering and measuring information on variables of interest.
Example: A researcher using surveys to collect feedback from customers about a product.
2. What are the primary data collection methods?
Primary data collection methods include surveys, interviews, observations, and focus groups, where data is gathered directly from the source.
3. What are secondary data collection methods?
Secondary methods involve collecting existing data from sources like government reports, company records, websites, or academic research papers.
4. What are quantitative and qualitative data collection methods?
Quantitative methods focus on numbers and statistics (e.g., surveys), while qualitative methods explore insights and opinions (e.g., interviews, focus groups).
5. What are data collection tools?
Tools used include SurveyMonkey, Google Forms, SurveyCTO, KoBoToolbox, Excel, SPSS, and mobile data collection apps.
6. Why is data collection important?
It helps in informed decision-making, trend analysis, strategy building, and measuring performance in business, research, and governance.
7. What industries rely heavily on data collection?
Industries like healthcare, education, marketing, finance, e-commerce, and public policy depend on accurate data collection for success.
8. What are common challenges in data collection?
Challenges include poor data quality, incomplete responses, bias, technical issues, and data privacy concerns.
9. What’s the difference between surveys and questionnaires?
Surveys are broader and may involve various methods, while questionnaires are structured sets of questions, typically used within surveys.
10. How do you ensure data quality?
Use clear instructions, test instruments, train data collectors, and implement data validation techniques during and after collection.
11. What is mobile data collection?
It involves using mobile devices (phones/tablets) to gather data in the field using apps like SurveyCTO, ODK, or KoBoToolbox.
12. What is the role of sampling in data collection?
Sampling involves selecting a subset of a population to represent the whole, making data collection more practical and cost-effective.
13. How do you choose the right data collection method?
Base your choice on your research goals, available resources, target audience, and whether you need qualitative or quantitative data.
14. Can data collection be done offline?
Yes, tools like ODK or KoBoToolbox support offline data collection and sync data once internet is available.
15. What are ethical considerations in data collection?
Include informed consent, privacy protection, confidentiality, and using data only for its intended purpose.